Predictive Analytics for Maintaining Power System Stability in Smart Energy Communities
Keywords:
digitalization, energy supply, emerging power grids, smart grids, power system stability, energy forecastingSynopsis
Digitalization and decentralization of energy supply have introduced several challenges to emerging power grids known as smart grids. One of the significant challenges, on the demand side, is preserving the stability of the power systems due to locally distributed energy sources such as micro-power generation and storage units among energy prosumers at the household and community levels. In this context, energy prosumers are defined as energy consumers who also generate, store and trade energy. Accurate predictions of energy supply and electric demand of prosuemrs can address the stability issues at local levels. This study aims to develop appropriate forecasting frameworks for such environments to preserve power stability.
Building on existing work on energy forecasting at low-aggregated levels, it asks: What factors influence most on consumption and generation patterns of residential customers as energy prosumers. It also investigates how the accuracy of forecasting models at the household and community levels can be improved.
Based on a review of the literature on energy forecasting and per- forming empirical study on real datasets, the forecasting frameworks were developed focusing on short-term prediction horizons. These frameworks are built upon predictive analytics including data col- lection, data analysis, data preprocessing, and predictive machine learning algorithms based on statistical learning, artificial neural networks and deep learning.
Analysis of experimental results demonstrated that load observa- tions from previous hours (lagged loads) along with air temperature and time variables highly affects the households’ consumption and generation behaviour. The results also indicate that the prediction accuracy of adopted machine learning techniques can be improved by feeding them with highly influential variables and appliance-level data as well as by combining multiple learning algorithms ranging from conventional to deep neural networks. Further research is needed to investigate online approaches that could strengthen the effectiveness of forecasting in time-sensitive energy environments.
References
[2] Damir Novosel Michael I. Henderson and Mariesa L. Crow. Electric Power Grid Modernization, Trends, Challenges and Opportunities. url: https : / /www.cmu.edu/epp/irle/readings/henderson-novosel-crow-electric-power-grid-modernization.pdf (visited on 11/01/2017).
[3] Stephen Khan. Transmission Loss. url: https://theconversation.com/we-calculated-emissions-due-to-electricity-loss-on-the-power-grid-globally-its-a-lot-128296 (visited on 12/01/2020).
[4] United States Environmental Protection Agency. Energy and the Environment. url: https :/ / www .epa .gov / energy / centralized-generation-electricity-and-its-impacts- environment (visited on 03/13/2018).
[5] Guobin Xu, Wei Yu, David Griffith, Nada Golmie, and Paul Moulema. "Toward integrating distributed energy resources and storage devices in smart grid." In: IEEE internet of things journal 4.1 (2016), pp. 192-204.
https://doi.org/10.1109/JIOT.2016.2640563
[6] AJ Dinusha Rathnayaka, Vidyasagar M Potdar, Tharam Dil- lon, and Samitha Kuruppu. "Framework to manage multiple goals in community-based energy sharing network in smart grid." In: International Journal of Electrical Power and Energy Systems 73 (2015), pp. 615-624.
https://doi.org/10.1016/j.ijepes.2015.05.008
[7] Verena Heinisch, Mikael Odenberger, Lisa Go¨ransson, and Filip Johnsson. "Organizing prosumers into electricity trading communities: Costs to attain electricity transfer limitations and self-sufficiency goals." In: International Journal of Energy Research 43.13 (2019), pp. 7021-7039.
https://doi.org/10.1002/er.4720
[8] Eunice Espe, Vidyasagar Potdar, and Elizabeth Chang. "Pro- sumer communities and relationships in smart grids: A liter- ature review, evolution and future directions." In: Energies 11.10 (2018), p. 2528.
https://doi.org/10.3390/en11102528
[9] Lulu Wen, Kaile Zhou, Shanlin Yang, and Xinhui Lu."Optimal load dispatch of community microgrid with deep learning based solar power and load forecasting." In: Energy 171 (2019), pp. 1053-1065.
https://doi.org/10.1016/j.energy.2019.01.075
[10] V Lavanya and N Senthil Kumar. "A review: control strategies for power quality improvement in microgrid." In: Int J Renew Energy Res 8 (2018), pp. 150-65.
[11] Luis Hernandez, Carlos Baladron, Javier M Aguiar, Bel'en Carro, Antonio J Sanchez-Esguevillas, Jaime Lloret, and Joaquim Massana. "A survey on electric power demand forecasting: fu- ture trends in smart grids, microgrids and smart buildings." In: IEEE Communications Surveys & Tutorials 16.3 (2014), pp. 1460-1495.
https://doi.org/10.1109/SURV.2014.032014.00094
[12] G Peter Zhang."Time series forecasting using a hybrid ARIMA and neural network model." In: Neurocomputing 50 (2003), pp. 159-175.
https://doi.org/10.1016/S0925-2312(01)00702-0
[13] Filipe Rodrigues, Carlos Cardeira, and Calado. "The Daily and Hourly Energy Consumption and Load Forecasting Using ANN Method: A Case Study Using a Set of 93 Households in Portugal." In: Energy Procedia 62 (2014), pp. 220-229.
https://doi.org/10.1016/j.egypro.2014.12.383
[14] Elena Mocanu, Phuong H Nguyen, Madeleine Gibescu, and Wil L Kling. "Comparison of machine learning methods for estimating energy consumption in buildings." In: 2014 inter- national conference on probabilistic methods applied to power systems (PMAPS). IEEE. 2014, pp. 1-6.
https://doi.org/10.1109/PMAPS.2014.6960635
[15] Aoife M Foley, Paul G Leahy, Antonino Marvuglia, and Eamon J McKeogh. "Current methods and advances in forecasting of wind power generation." In: Renewable Energy 37.1 (2012), pp. 1-8.
https://doi.org/10.1016/j.renene.2011.05.033
[16] Cristian-Dragos Dumitru and Adrian Gligor. "Daily Average Wind Energy Forecasting Using Artificial Neural Networks." In: Procedia Engineering 181 (2017), pp. 829-836. issn: 1877-7058.
https://doi.org/10.1016/j.proeng.2017.02.474
[17] Sthitapragyan Mohanty, Prashanta K. Patra, Sudhansu S. Sahoo, and Asit Mohanty. "Forecasting of solar energy with application for a growing economy like India: Survey and implication." In: Renewable and Sustainable Energy Reviews 78 (2017), pp. 539-553. issn: 1364-0321. url: http://www.sciencedirect.com/science/article/pii/S1364032117305853.
https://doi.org/10.1016/j.rser.2017.04.107
[18] Abinet Tesfaye Eseye, Jianhua Zhang, and Dehua Zheng. "Short-term photovoltaic solar power forecasting using a hybrid Wavelet-PSO-SVM model based on SCADA and Meteorologi- cal information." In: Renewable Energy 118 (2018), pp. 357-367. issn: 0960-1481. url: http://www.sciencedirect. com/science/article/pii/S0960148117311126.
https://doi.org/10.1016/j.renene.2017.11.011
[19] Cyril Voyant, Ted Soubdhan, Philippe Lauret, Mathieu David, and Marc Muselli. "Statistical parameters as a means to a priori assess the accuracy of solar forecasting models." In: Energy 90 (2015), pp. 671-679.
https://doi.org/10.1016/j.energy.2015.07.089
[20] A. M. Pirbazari, A. Chakravorty, and C. Rong. "Evaluating Feature Selection Methods for Short-Term Load Forecasting." In: 2019 IEEE International Conference on Big Data and Smart Computing (BigComp). 2019, pp. 1-8.
https://doi.org/10.1109/BIGCOMP.2019.8679188
[21] Aida Mehdipour Pirbazari, Mina Farmanbar, Antorweep Chakra- vorty, and Chunming Rong. "Short-Term Load Forecasting Using Smart Meter Data: A Generalization Analysis." In: Pro- cesses 8.4 (2020). issn: 2227-9717. url: https://www.mdpi. com/2227-9717/8/4/484.
https://doi.org/10.3390/pr8040484
[22] A. M. Pirbazari, M. Farmanbar, A. Chakravorty, and C. Rong. "Improving Load Forecast Accuracy of Households Us- ing Load Disaggregation Techniques." In: 2020 International Conferences on Internet of Things (iThings) and IEEE Green Computing and Communications (GreenCom) and IEEE Cy- ber, Physical and Social Computing (CPSCom) and IEEE Smart Data (SmartData) and IEEE Congress on Cybermatics (Cybermatics). 2020, pp. 843-851.
https://doi.org/10.1109/iThings-GreenCom-CPSCom-SmartData-Cybermatics50389.2020.00140
[23] Tao Hong et al. "Energy forecasting: Past, present, and future." In: Foresight: The International Journal of Applied Forecasting 32 (2014), pp. 43-48.
[24] Henrique Steinherz Hippert, Carlos Eduardo Pedreira, and Reinaldo Castro Souza. "Neural networks for short-term load forecasting: A review and evaluation." In: IEEE Transactions on power systems 16.1 (2001), pp. 44-55.
https://doi.org/10.1109/59.910780
[25] L. Ghods and M. Kalantar. "Methods for long-term electric load demand forecasting; a comprehensive investigation." In: 2008 IEEE International Conference on Industrial Technology. 2008, pp. 1-4.
https://doi.org/10.1109/ICIT.2008.4608469
[26] Elena Mocanu, Phuong H Nguyen, Madeleine Gibescu, and Wil L Kling. "Deep learning for estimating building energy consumption." In: Sustainable Energy, Grids and Networks 6 (2016), pp. 91-99.
https://doi.org/10.1016/j.segan.2016.02.005
[27] Chun-Nam Yu, Piotr Mirowski, and Tin Kam Ho. "A sparse coding approach to household electricity demand forecasting in smart grids." In: IEEE Transactions on Smart Grid 8.2 (2016), pp. 738-748.
[28] Rodrigo F Berriel, Andre Teixeira Lopes, Alexandre Rodrigues, Flavio Miguel Varejao, and Thiago Oliveira-Santos. "Monthly energy consumption forecast: A deep learning approach." In: 2017 International Joint Conference on Neural Networks (IJCNN). IEEE. 2017, pp. 4283-4290.
https://doi.org/10.1109/IJCNN.2017.7966398
[29] Xueheng Qiu, Ye Ren, Ponnuthurai Nagaratnam Suganthan, and Gehan AJ Amaratunga. "Empirical mode decomposition based ensemble deep learning for load demand time seriesforecasting." In: Applied Soft Computing 54 (2017), pp. 246-255.
https://doi.org/10.1016/j.asoc.2017.01.015
[30] Vasudev Dehalwar, Akhtar Kalam, Mohan Lal Kolhe, and Al- adin Zayegh. "Electricity load forecasting for Urban area using weather forecast information." In: 2016 IEEE International Conference on Power and Renewable Energy (ICPRE). IEEE. 2016, pp. 355-359.
https://doi.org/10.1109/ICPRE.2016.7871231
[31] Magnus Dahl, Adam Brun, Oliver S Kirsebom, and Gorm B Andresen. "Improving short-term heat load forecasts with calendar and holiday data." In: Energies 11.7 (2018), p. 1678.
https://doi.org/10.3390/en11071678
[32] Franklin L Quilumba, Wei-Jen Lee, Heng Huang, David Y Wang, and Robert L Szabados. "Using smart meter data to improve the accuracy of intraday load forecasting considering customer behavior similarities." In: IEEE Transactions on Smart Grid 6.2 (2014), pp. 911-918.
https://doi.org/10.1109/TSG.2014.2364233
[33] Min Han and Y Wang. "Analysis and modeling of multivari- ate chaotic time series based on neural network." In: Expert Systems with Applications 36.2 (2009), pp. 1280-1290.
https://doi.org/10.1016/j.eswa.2007.11.057
[34] George Atsalakis and Katerina Tsakalaki. "Simulating anneal- ing and neural networks for chaotic time series forecasting." In: Chaotic Modeling and Simulation 1 (2012), pp. 81-90.
[35] Matteo Sangiorgio and Fabio Dercole. "Robustness of LSTM neural networks for multi-step forecasting of chaotic time series." In: Chaos, Solitons & Fractals 139 (2020), p. 110045.
https://doi.org/10.1016/j.chaos.2020.110045
[36] Gianluca Bontempi, Souhaib Ben Taieb, and Yann-A¨el Le Borgne. "Machine Learning Strategies for Time Series Fore- casting." In: Business Intelligence: Second European Summer School, eBISS 2012, Brussels, Belgium, July 15-21, 2012, Tuto- rial Lectures. Ed. by Marie-Aude Aufaure and Esteban Zima'nyi. Springer Berlin Heidelberg, 2013, pp. 62-77.
[37] Tao Hong and Shu Fan. "Probabilistic electric load forecasting: A tutorial review." In: International Journal of Forecasting 32.3 (2016), pp. 914-938.
https://doi.org/10.1016/j.ijforecast.2015.11.011
[38] Zeyu Wang, Yueren Wang, and Ravi S Srinivasan. "A novel ensemble learning approach to support building energy use prediction." In: Energy and Buildings 159 (2018), pp. 109-122.
https://doi.org/10.1016/j.enbuild.2017.10.085
[39] Arjun Baliyan, Kumar Gaurav, and Sudhansu Kumar Mishra. "A review of short term load forecasting using artificial neural network models." In: Procedia Computer Science 48 (2015), pp. 121-125.
https://doi.org/10.1016/j.procs.2015.04.160
[40] Hamid R Khosravani, Marıa Del Mar Castilla, Manuel Berenguel, Antonio E Ruano, and Pedro M Ferreira. "A comparison of en- ergy consumption prediction models based on neural networks of a bioclimatic building." In: Energies 9.1 (2016), p. 57.
https://doi.org/10.3390/en9010057
[41] Ahmad S Ahmad, Mohammad Y Hassan, Md P Abdullah, Hasimah A Rahman, F Hussin, Hayati Abdullah, and Rahman Saidur. "A review on applications of ANN and SVM for build- ing electrical energy consumption forecasting." In: Renewable and Sustainable Energy Reviews 33 (2014), pp. 102-109.
https://doi.org/10.1016/j.rser.2014.01.069
[42] Fateme Fahiman, Sarah M Erfani, Sutharshan Rajasegarar, Marimuthu Palaniswami, and Christopher Leckie. "Improving load forecasting based on deep learning and K-shape clus- tering." In: 2017 International Joint Conference on Neural Networks (IJCNN). IEEE. 2017, pp. 4134-4141.
https://doi.org/10.1109/IJCNN.2017.7966378
[43] Weicong Kong, Zhao Yang Dong, Youwei Jia, David J Hill, Yan Xu, and Yuan Zhang. "Short-term residential load fore- casting based on LSTM recurrent neural network." In: IEEE Transactions on Smart Grid 10.1 (2017), pp. 841-851.
https://doi.org/10.1109/TSG.2017.2753802
[44] Mengmeng Cai, Manisa Pipattanasomporn, and Saifur Rah- man. "Day-ahead building-level load forecasts using deep learn- ing vs. traditional time-series techniques." In: Applied Energy 236 (2019), pp. 1078-1088.
https://doi.org/10.1016/j.apenergy.2018.12.042
[45] Abdulaziz Almalaq and George Edwards. "A review of deep learning methods applied on load forecasting." In: 2017 16th IEEE international conference on machine learning and appli- cations (ICMLA). IEEE. 2017, pp. 511-516.
https://doi.org/10.1109/ICMLA.2017.0-110
[46] Jinliang Zhang, Yi-Ming Wei, Dezhi Li, Zhongfu Tan, and Jianhua Zhou. "Short term electricity load forecasting using a hybrid model." In: Energy 158 (2018), pp. 774-781.
https://doi.org/10.1016/j.energy.2018.06.012
[47] Yanbing Lin, Hongyuan Luo, Deyun Wang, Haixiang Guo, and Kejun Zhu. "An ensemble model based on machine learning methods and data preprocessing for short-term electric load forecasting." In: Energies 10.8 (2017), p. 1186.
https://doi.org/10.3390/en10081186
[48] Wei Tang Zhi Zhou Wu. "Ensembling neural networks, Many could be better than all." In: Artificial Intelligence 137 (2002), pp. 239-263.
https://doi.org/10.1016/S0004-3702(02)00190-X
[49] Xueheng Qiu, Le Zhang, Ye Ren, Ponnuthurai N Suganthan, and Gehan Amaratunga. "Ensemble deep learning for regres- sion and time series forecasting." In: Computational Intelli- gence in Ensemble Learning (CIEL), 2014 IEEE Symposium on. IEEE, 2014, pp. 1-6.
[50] IRENA. url: https://www.irena.org/Statistics/View- Data-by-Topic/Capacity-and-Generation/Technologies (visited on 12/01/2020).
[51] H. Wang, J. Ruan, G. Wang, B. Zhou, Y. Liu, X. Fu, and J. Peng. "Deep Learning-Based Interval State Estimation of AC Smart Grids Against Sparse Cyber Attacks." In: IEEE Transactions on Industrial Informatics 14.11 (2018), pp. 4766- 4778.
https://doi.org/10.1109/TII.2018.2804669
[52] Huaizhi Wang, Zhenxing Lei, Xian Zhang, Bin Zhou, and Jianchun Peng."A review of deep learning for renewable energy forecasting." In: Energy Conversion and Management 198 (2019), p. 111799.
https://doi.org/10.1016/j.enconman.2019.111799
[53] Sultan Al-Yahyai, Yassine Charabi, and Adel Gastli. "Review of the use of numerical weather prediction (NWP) models for wind energy assessment." In: Renewable and Sustainable Energy Reviews 14.9 (2010), pp. 3192-3198.
https://doi.org/10.1016/j.rser.2010.07.001
[54] David P Larson, Lukas Nonnenmacher, and Carlos FM Coim- bra. "Day-ahead forecasting of solar power output from pho- tovoltaic plants in the American Southwest." In: Renewable Energy 91 (2016), pp. 11-20.
https://doi.org/10.1016/j.renene.2016.01.039
[55] Bri-Mathias Hodge, Carlo Brancucci Martinez-Anido, Qin Wang, Erol Chartan, Anthony Florita, and Juha Kiviluoma. "The combined value of wind and solar power forecasting improvements and electricity storage." In: Applied Energy 214 (2018), pp. 1-15.
https://doi.org/10.1016/j.apenergy.2017.12.120
[56] Stefano Ferrari, Massimo Lazzaroni, Vincenzo Piuri, Loredana Cristaldi, and Marco Faifer. "Statistical models approach for solar radiation prediction." In: 2013 IEEE international instru- mentation and measurement technology conference (I2MTC). IEEE. 2013, pp. 1734-1739.
https://doi.org/10.1109/I2MTC.2013.6555712
[57] Rui Huang, Tiana Huang, Rajit Gadh, and Na Li."Solar gener- ation prediction using the ARMA model in a laboratory-level micro-grid." In: 2012 IEEE third international conference on smart grid communications (SmartGridComm). IEEE. 2012, pp. 528-533.
https://doi.org/10.1109/SmartGridComm.2012.6486039
[58] SN Singh, Abheejeet Mohapatra, et al. "Repeated wavelet transform based ARIMA model for very short-term wind speed forecasting." In: Renewable energy 136 (2019), pp. 758-768.
https://doi.org/10.1016/j.renene.2019.01.031
[59] Ilhami Colak, Mehmet Yesilbudak, Naci Genc, and Ramazan Bayindir. "Multi-period prediction of solar radiation using ARMA and ARIMA models." In: 2015 IEEE 14th international conference on machine learning and applications (ICMLA). IEEE. 2015, pp. 1045-1049.
https://doi.org/10.1109/ICMLA.2015.33
[60] Yun Wang, Haibo Wang, Dipti Srinivasan, and Qinghua Hu. "Robust functional regression for wind speed forecasting based on Sparse Bayesian learning." In: Renewable Energy 132 (2019), pp. 43-60.
https://doi.org/10.1016/j.renene.2018.07.083
[61] Jianwu Zeng and Wei Qiao. "Short-term solar power prediction using a support vector machine." In: Renewable Energy 52 (2013), pp. 118-127.
https://doi.org/10.1016/j.renene.2012.10.009
[62] Jianzhou Wang, Na Zhang, and Haiyan Lu. "A novel system based on neural networks with linear combination framework for wind speed forecasting." In: Energy Conversion and Man- agement 181 (2019), pp. 425-442.
https://doi.org/10.1016/j.enconman.2018.12.020
[63] Amir Sharifian, M Jabbari Ghadi, Sahand Ghavidel, Li Li, and Jiangfeng Zhang."A new method based on Type-2 fuzzy neural network for accurate wind power forecasting under uncertain data." In: Renewable energy 120 (2018), pp. 220-230.
https://doi.org/10.1016/j.renene.2017.12.023
[64] Woonghee Lee, Keonwoo Kim, Junsep Park, Jinhee Kim, and Younghoon Kim. "Forecasting solar power using long-short term memory and convolutional neural networks." In: IEEE Access 6 (2018), pp. 73068-73080.
https://doi.org/10.1109/ACCESS.2018.2883330
[65] Andr'e Gensler, Janosch Henze, Bernhard Sick, and Nils Raabe. "Deep Learning for solar power forecasting-An approach using AutoEncoder and LSTM Neural Networks." In: 2016 IEEE international conference on systems, man, and cybernetics (SMC). IEEE. 2016, pp. 002858-002865.
https://doi.org/10.1109/SMC.2016.7844673
[66] Chun-Yang Zhang, CL Philip Chen, Min Gan, and Long Chen. "Predictive deep Boltzmann machine for multiperiod wind speed forecasting." In: IEEE Transactions on Sustainable En- ergy 6.4 (2015), pp. 1416-1425.
https://doi.org/10.1109/TSTE.2015.2434387
[67] Huaizhi Wang, Haiyan Yi, Jianchun Peng, Guibin Wang, Yitao Liu, Hui Jiang, and Wenxin Liu. "Deterministic and probabilis- tic forecasting of photovoltaic power based on deep convolu- tional neural network." In: Energy conversion and management 153 (2017), pp. 409-422.
https://doi.org/10.1016/j.enconman.2017.10.008
[68] Yanfei Li, Huipeng Shi, Fengze Han, Zhu Duan, and Hui Liu. "Smart wind speed forecasting approach using various boosting algorithms, big multi-step forecasting strategy." In: Renewable energy 135 (2019), pp. 540-553.
https://doi.org/10.1016/j.renene.2018.12.035
[69] Azhar Ahmed Mohammed, Waheeb Yaqub, and Zeyar Aung. "Probabilistic forecasting of solar power: An ensemble learning approach." In: International Conference on Intelligent Decision Technologies. Springer. 2017, pp. 449-458.
https://doi.org/10.1007/978-3-319-19857-6_38
[70] Ye Ren, PN Suganthan, and N Srikanth. "Ensemble meth- ods for wind and solar power forecasting-A state-of-the-art review." In: Renewable and Sustainable Energy Reviews 50 (2015), pp. 82-91.
https://doi.org/10.1016/j.rser.2015.04.081
[71] Antorweep Chakravorty, Chunming Rong, P˚al Evensen, and Tomasz Wiktor Wlodarczyk. "A distributed gaussian-means clustering algorithm for forecasting domestic energy usage." In: 2014 International Conference on Smart Computing. IEEE. 2014, pp. 229-236.
https://doi.org/10.1109/SMARTCOMP.2014.7043863
[72] Tom M Mitchell. "Artificial neural networks." In: Machine learning 45 (1997), pp. 81-127.
[73] Isabelle Guyon and Andr'e Elisseeff. "An introduction to vari- able and feature selection." In: Journal of machine learning research 3.Mar (2003), pp. 1157-1182.
[74] Sanghamitra Bandyopadhyay and Sriparna Saha. Unsupervised classification: similarity measures, classical and metaheuristic approaches, and applications. Springer Science & Business Media, 2012.
https://doi.org/10.1007/978-3-642-32451-2_3
[75] Ali Al-Wakeel, Jianzhong Wu, and Nick Jenkins. "K-means based load estimation of domestic smart meter measurements." In: Applied energy 194 (2017), pp. 333-342.
https://doi.org/10.1016/j.apenergy.2016.06.046
[76] Fintan McLoughlin, Aidan Duffy, and Michael Conlon. "A clustering approach to domestic electricity load profile charac- terisation using smart metering data." In: Applied energy 141 (2015), pp. 190-199.
https://doi.org/10.1016/j.apenergy.2014.12.039
[77] Joshua D Rhodes, Wesley J Cole, Charles R Upshaw, Thomas F Edgar, and Michael E Webber. "Clustering analysis of res- idential electricity demand profiles." In: Applied Energy 135 (2014), pp. 461-471.
https://doi.org/10.1016/j.apenergy.2014.08.111
[78] James Durbin and Siem Jan Koopman. Time series analysis by state space methods. Oxford University Press, 2012.
https://doi.org/10.1093/acprof:oso/9780199641178.001.0001
[79] Hongzhan Nie, Guohui Liu, Xiaoman Liu, and Yong Wang. "Hybrid of ARIMA and SVMs for short-term load forecasting." In: Energy Procedia 16 (2012), pp. 1455-1460.
https://doi.org/10.1016/j.egypro.2012.01.229
[80] Sharif Atique, Subrina Noureen, Vishwajit Roy, Vinitha Sub- buraj, Stephen Bayne, and Joshua Macfie."Forecasting of total daily solar energy generation using ARIMA: A case study." In: 2019 IEEE 9th annual computing and communication work- shop and conference (CCWC). IEEE. 2019, pp. 0114-0119.
https://doi.org/10.1109/CCWC.2019.8666481
[81] Arthur E Hoerl and Robert W Kennard. "Ridge regression: Biased estimation for nonorthogonal problems." In: Techno- metrics 12.1 (1970), pp. 55-67.
https://doi.org/10.1080/00401706.1970.10488634
[82] AK Md Ehsanes Saleh, Mohammad Arashi, and BM Golam Kibria. Theory of ridge regression estimation with applications. Vol. 285. John Wiley & Sons, 2019.
[83] Nello Cristianini, John Shawe-Taylor, et al. An introduction to support vector machines and other kernel-based learning methods. Cambridge university press, 2000.
https://doi.org/10.1017/CBO9780511801389
[84] Zhi-Hua Zhou. Ensemble methods: foundations and algorithms. CRC press, 2012.
https://doi.org/10.1201/b12207
[85] Leo Breiman. "Random forests." In: Machine learning 45.1 (2001), pp. 5-32.
https://doi.org/10.1023/A:1010933404324
[86] Leo Breiman, Jerome Friedman, Charles J Stone, and Richard A Olshen. Classification and regression trees. CRC press, 1984.
[87] Devon K Barrow and Sven F Crone. "A comparison of Ad- aBoost algorithms for time series forecast combination." In: International Journal of Forecasting 32.4 (2016), pp. 1103- 1119.
https://doi.org/10.1016/j.ijforecast.2016.01.006
[88] Jerome H Friedman. "Greedy function approximation: a gradi- ent boosting machine." In: Annals of statistics (2001), pp. 1189- 1232.
https://doi.org/10.1214/aos/1013203451
[89] Jesu's Ferrero Bermejo, Juan F Gomez Fernandez, Fernando Olivencia Polo, and Adolfo Crespo Marquez. "A review of the use of artificial neural network models for energy and reliability prediction. A study of the solar PV, hydraulic and wind energy sources." In: Applied Sciences 9.9 (2019), p. 1844.
https://doi.org/10.3390/app9091844
[90] Zheng Yi. "Evaluation and implementation of convolutional neural networks in image recognition." In: Journal of Physics: Conference Series. Vol. 1087. 6. IOP Publishing. 2018, p. 062018.
https://doi.org/10.1088/1742-6596/1087/6/062018
[91] Mahbub Hussain, Jordan J Bird, and Diego R Faria. "A study on cnn transfer learning for image classification." In: UK Work- shop on computational Intelligence. Springer. 2018, pp. 191- 202.
https://doi.org/10.1007/978-3-319-97982-3_16
[92] Irena Koprinska, Dengsong Wu, and Zheng Wang. "Convo- lutional neural networks for energy time series forecasting." In: 2018 international joint conference on neural networks (IJCNN). IEEE. 2018, pp. 1-8.
https://doi.org/10.1109/IJCNN.2018.8489399
[93] Kasun Amarasinghe, Daniel L Marino, and Milos Manic."Deep neural networks for energy load forecasting." In: 2017 IEEE 26th International Symposium on Industrial Electronics (ISIE). IEEE. 2017, pp. 1483-1488.
https://doi.org/10.1109/ISIE.2017.8001465
[94] Sepp Hochreiter and Ju¨rgen Schmidhuber. "Long short-term memory." In: Neural computation 9.8 (1997), pp. 1735-1780.
https://doi.org/10.1162/neco.1997.9.8.1735
[95] Daniel L Marino, Kasun Amarasinghe, and Milos Manic. "Building energy load forecasting using deep neural networks." In: IECON 2016-42nd Annual Conference of the IEEE Indus- trial Electronics Society. IEEE. 2016, pp. 7046-7051.
https://doi.org/10.1109/IECON.2016.7793413
[96] Ilya Sutskever, Oriol Vinyals, and Quoc V Le. "Sequence to sequence learning with neural networks." In: arXiv preprint arXiv:1409.3215 (2014).
[97] Alex Graves and Navdeep Jaitly. "Towards end-to-end speech recognition with recurrent neural networks." In: International conference on machine learning. PMLR. 2014, pp. 1764-1772.
[98] Kyunghyun Cho, Bart Van Merri¨enboer, Dzmitry Bahdanau, and Yoshua Bengio. "On the properties of neural machine translation: Encoder-decoder approaches." In: arXiv preprint arXiv:1409.1259 (2014).
[99] Junyoung Chung, Caglar Gulcehre, KyungHyun Cho, and Yoshua Bengio."Empirical evaluation of gated recurrent neural networks on sequence modeling." In: arXiv preprint arXiv:1412.3555 (2014).
[100] Mirco Ravanelli, Philemon Brakel, Maurizio Omologo, and Yoshua Bengio. "Light gated recurrent units for speech recog- nition." In: IEEE Transactions on Emerging Topics in Com- putational Intelligence 2.2 (2018), pp. 92-102.
https://doi.org/10.1109/TETCI.2017.2762739
[101] Muhammad Sajjad, Zulfiqar Ahmad Khan, Amin Ullah, Tan- veer Hussain, Waseem Ullah, Mi Young Lee, and Sung Wook Baik. "A novel CNN-GRU-based hybrid approach for short- term residential load forecasting." In: IEEE Access 8 (2020), pp. 143759-143768.
https://doi.org/10.1109/ACCESS.2020.3009537
[102] Yusen Wang, Wenlong Liao, and Yuqing Chang. "Gated recur- rent unit network-based short-term photovoltaic forecasting." In: Energies 11.8 (2018), p. 2163.
https://doi.org/10.3390/en11082163
[103] Pankaj Malhotra, Anusha Ramakrishnan, Gaurangi Anand, Lovekesh Vig, Puneet Agarwal, and Gautam Shroff. "LSTM- based encoder-decoder for multi-sensor anomaly detection." In: arXiv preprint arXiv:1607.00148 (2016).
[104] Shengdong Du, Tianrui Li, Yan Yang, and Shi-Jinn Horng. "Multivariate time series forecasting via attention-based encoder- decoder framework." In: Neurocomputing 388 (2020), pp. 269- 279.
https://doi.org/10.1016/j.neucom.2019.12.118
[105] Wan He and Ying Chai. "An empirical study on energy dis- aggregation via deep learning." In: 2016 2nd International Conference on Artificial Intelligence and Industrial Engineer- ing (AIIE 2016). Atlantis Press. 2016.
https://doi.org/10.2991/aiie-16.2016.77
[106] Bin Zhou, Wentao Li, K.W. Chan, Yijia Cao, Yonghong Kuang, Xi Liu, and Xiong Wang. "Smart home energy management systems: Concept, configurations, and scheduling strategies." In: Renewable and Sustainable Energy Reviews 61 (Aug. 2016), pp. 30-40.
https://doi.org/10.1016/j.rser.2016.03.047
[107] Felan Carlo C Garcia, Christine May C Creayla, and Erees Queen B Macabebe. "Development of an intelligent system for smart home energy disaggregation using stacked denoising autoencoders." In: Procedia Computer Science 105 (2017), pp. 248-255.
https://doi.org/10.1016/j.procs.2017.01.218
[108] Anthony Faustine, Nerey Henry Mvungi, Shubi Kaijage, and Kisangiri Michael. "A survey on non-intrusive load monitoring methodies and techniques for energy disaggregation problem." In: arXiv preprint arXiv:1703.00785 (2017).
[109] I Abubakar, SN Khalid, MW Mustafa, Hussain Shareef, and M Mustapha. "Application of load monitoring in appliances' energy management-A review." In: Renewable and Sustainable Energy Reviews 67 (2017), pp. 235-245.
https://doi.org/10.1016/j.rser.2016.09.064
[110] G. A. Raiker, S. B. Reddy, L. Umanand, A. Yadav, and M. M. Shaikh. "Approach to Non-Intrusive Load Monitoring using Factorial Hidden Markov Model." In: 2018 IEEE 13th Inter- national Conference on Industrial and Information Systems (ICIIS). 2018, pp. 381-386.
https://doi.org/10.1109/ICIINFS.2018.8721436
[111] Ahmed Zoha, Alexander Gluhak, Michele Nati, and Muham- mad Ali Imran. "Low-power appliance monitoring using fac- torial hidden markov models." In: 2013 IEEE Eighth Inter- national Conference on Intelligent Sensors, Sensor Networks and Information Processing. IEEE. 2013, pp. 527-532.
https://doi.org/10.1109/ISSNIP.2013.6529845
[112] Jack Kelly and William Knottenbelt. "Neural nilm: Deep neu- ral networks applied to energy disaggregation." In: Proceedings of the 2nd ACM international conference on embedded systems for energy-efficient built environments. 2015, pp. 55-64.
https://doi.org/10.1145/2821650.2821672
[113] Chaoyun Zhang, Mingjun Zhong, Zongzuo Wang, Nigel God- dard, and Charles Sutton. "Sequence-to-point learning with neural networks for non-intrusive load monitoring." In: Pro- ceedings of the AAAI Conference on Artificial Intelligence. Vol. 32. 1. 2018.
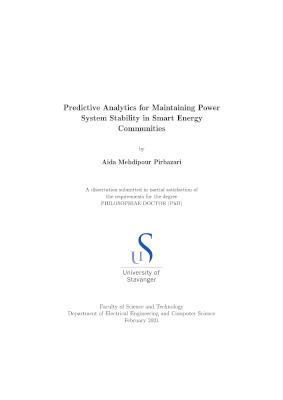