Methodological developments for ensemble-based optimization of EOR processes
Keywords:
The National IOR Centre of Norway, EOR, ensemble-based optimization, waterfloodingSynopsis
Ensemble-based tools for production optimization has been developed over the last couple of decades, with a special focus on waterflooding. In our setting it will be important to expand this to include cases covering injection of smart water, CO2 and polymer. Environmental constraints, including energy eciency, should be added in the objective function. When considering implementation of any IOR or EOR method on a real eld, it is of outermost importance to be able to demonstrate the potential, with as accurate as possible uncertainty quantication. Ensemble-based optimization has the potential to handle this as it can include all sources of uncertainties in the ensemble of reservoir models (also referred to as geological realizations).
In this document we will present ensemble-based optimization of waterflooding and EOR processes (CO2, polymer and smart water). The optimization is performed to achieve a maximum net-present-value (NPV) for a given reservoir. The uncertainty in the reservoir description is included by providing an ensemble of reservoir models where important reservoir parameters (as porosity, permeability, etc.) varies. Economic input parameters includes oil price and cost of water injection and production as well as cost related to injection and production of EOR chemicals. In addition, a discount rate is included, but uncertainties in
economical parameters are excluded.
The methodological development described in this document does not depend on any particular properties of the reservoir. However, for better illustration, two synthetic elds are chosen as demonstration cases. The two example cases are the OLYMPUS eld designed by TNO and the Reek eld designed by Equinor. The OLYMPUS eld is inspired and loosely based on Brent-type oil elds in the North Sea (Fonseca et al., 2018). The REEK eld is a reservoir model developed in Equinor and originally used as an internal benchmark model for testing of dierent algorithms and workflows (Hanea et al., 2017).
The choice of these two elds for the demonstration of the methodology is based on a balance between including realistic features in the model and having models where a large number of simulations can be performed with modest computational resources. The computational time for a simulation of a waterflooding process on the OLYMPUS eld is about 10 minutes. The computational time for actual eld cases might be larger, but one of the strengths of ensemble-based optimization is that many of the required simulations can be run in parallel. Still, a judgment of the required computational load prior to performing the
optimization will be important. For the methodological development that has been done as a part of the IOR center, it was preferable to work on cases with modest computational time, without sacricing to much of realism. The OLYMPUS and REEK elds where considered as good choices in this respect. Moreover, these elds have both been used in other studies,
making comparisons possible in some cases.
References
Y. Chen, D. S. Oliver, and D. Zhang. E cient ensemble-based closed-loop production optimization. SPE Journal, 14(04):634-645, 2009. https://doi.org/10.2118/112873-PA
ECLIPSE. Eclipse. https://www.software.slb.com/products/eclipse. Accessed: 2021-09-22.
R. M. Fonseca, B. Chen, J. D. Jansen, and A. Reynolds. A stochastic simplex approximate gradient (StoSAG) for optimization under uncertainty. International Journal for Numerical Methods in Engineering, 109(13):1756-1776, 2017.
https://doi.org/10.1002/nme.5342
R. M. Fonseca, E. D. Rossa, A. A. Emerick, R. G. Hanea, and J. D. Jansen. Overview of the olympus field development optimization challenge. In ECMOR XVI 2018 - 16th European Conference on the Mathematics of Oil Recovery. EAGE, September 3-6 2018.
https://doi.org/10.3997/2214-4609.201802246
R. Hanea, P. Casanova, F. H. Wilschut, and R. Fonseca. Well trajectory optimization constrained to structural uncertainties. In SPE Reservoir Simulation Conference. Society of Petroleum Engineers, 2017.
https://doi.org/10.2118/182680-MS
J. Nocedal and S. Wright. Numerical optimization. Springer Science & Business Media, 2006.
M. Oguntola and R. Lorentzen. On the robust value quantification of polymer EOR injection strategies for better decision making. In ECMOR XVII, volume 2020. European Association of Geoscientists & Engineers, 2020.
https://doi.org/10.3997/2214-4609.202035057
M. B. Oguntola and R. J. Lorentzen. Ensemble-based constrained optimization using an exterior penalty method. Journal of Petroleum Science and Engineering, 207:109165, 2021a. ISSN 0920-4105.
https://doi.org/10.1016/j.petrol.2021.109165
M. B. Oguntola and R. J. Lorentzen. Robust value quantification of enhanced oil recovery methods using ensemble-based optimization. Submitted to "SPE Reservoir Evaluation & Engineering", 2021b.
https://doi.org/10.2118/209587-PA
OPM. Open porus media. https://opm-project.org/. Accessed: 2021-09-22.
V. L. S. Silva, M. A. Cardoso, D. F. B. Oliveira, and R. J. de Moraes. Stochastic optimization strategies applied to the OLYMPUS benchmark. Computational Geosciences, Oct 2019.
https://doi.org/10.3997/2214-4609.201802289
A. Stordal, Y. Chang, R. Hanea, and T. Ek. Ensemble based optimization using importance sampling and gaussian mixtures. In ECMOR XV-15th European conference on the mathematics of oil recovery, 2016.
TNO. https://www.tno.nl/en/. Accessed: 2021-09-29.
TNO. OLYMPUS optimization challenge. http://www.isapp2.com/optimization-challenge/problem-statement.html, 2017. Accessed: 2021-09-22.
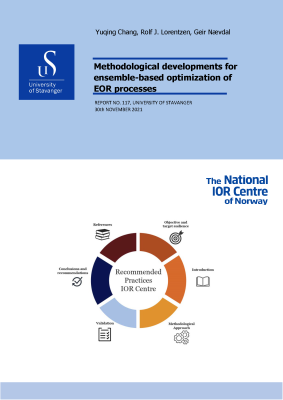