Human Capital Production in Childhood: Essays on the Economics of Education
Keywords:
econometrics, childhood skill formation, human capital production, economics of educationSynopsis
The skills demanded in the labor market have changed (see, e.g., Ace- moglu & Autor 2011).1 Just as technological innovations have increased demand for workers capable of performing non-routine and complex tasks, demand for routine work has declined (Autor et al. 2003, Spitz-Oener 2006). Since lifelong learning can help individuals develop resilience and adapt to adverse shocks in changing labor markets, it is important to learn more about the best ways to support such learning.2
Skill formation starts in childhood, as does lifelong learning. By in- vesting early, and with the support of teachers and caregivers, we can equip children with a strong foundation for further development (National Research Council Institute of Medicine 2000). Societies can invest in skill formation by improving the quality of children’s environments. Indeed, the home environment, early childhood education and care (ECEC) centers, and schools can all affect children’s skill formation (Almond & Currie 2011, Blau & Currie 2006, Cunha et al. 2006, Hanushek & Rivkin 2006, Heckman & Mosso 2014). This influential role of the environment has implications for policy because it suggests a role for investments that enhance its quality. Yet gathering evidence on environmental factors that affect skill formation is no simple task, and much remains to be learned about the relevant processes.
This thesis focuses on three key questions: First, what is the best way to gain a better understanding of how children acquire new skills? Econo- metric tools have been developed to evaluate the effectiveness of environ- mental factors that affect skill formation. However, these tools cannot be employed unless children are observed at equally spaced intervals. Second, which of the many possible skills should investments focus on? During early childhood, it is best to target skills that promote a child’s further development, and in the context of the crucial transition from preschool to primary school, these could be skills that help prepare children for success in school. Third, once certain skills have been nurtured during early child- hood, how can we provide effective education to sustain and build on this foundation? Although we know that teachers play a key role in education, much remains to be learned about what makes teachers effective. By ad- dressing these questions, this thesis seeks to deepen our understanding of the processes driving skill formation in childhood, yielding new and better insights into public policy design. The individual chapters are described in more detail below.
Chapter 2 examines the literature on the econometrics of childhood skill formation. This literature comprises econometric tools for assess- ing the effectiveness of environmental factors and models influences on skill formation. In particular, it helps us understand what can be learned from a model of skill formation (identification), how we can best learn it (estimation), and how certain we can be about parameters we estimate (inference). Todd & Wolpin (2003), Cunha & Heckman (2008), Cunha et al. (2010), and Agostinelli & Wiswall (2016) develop tools to address several challenges. First, scales used to assess children may not have a cardinal interpretation. Second, given the difficulty of assessing children, any instrument is likely to be subject to measurement error. Third, unob- served inputs may correlate with observed inputs. Just as non-cardinality may result in biased interpretation, measurement error and the presence of unobserved inputs may cause biased estimation.
I evaluate the implications of a challenge that has received little attentionin the past. When we estimate skill formation models, we typically assume that all children are observed in equally spaced intervals. The interval assumed by our model is therefore like the one observed in the data. In most longitudinal studies, however, observation intervals are not equally spaced (McKenzie 2001, Millimet & McDonough 2017). One might also argue that the substantive timing variable of child development is age, not the assessment wave. In this case, unequal spacing may occur even when survey waves are equidistant because (i) same-aged children may not be observed simultaneously, and (ii) simultaneously observed children may not be of the same age.
Most longitudinal studies target “same-aged” children based on birth year. The development of children born in the same year and assessed simultaneously can differ by as much as 12 months. If, in addition, as- sessments can occur at any time during the year, then the development of children born in the same year may differ by as much as 24 months. Such differences can have an impact on cognitive skill formation (Crawford et al. 2010). Of course, we would expect these developmental differences to become smaller as children grow older (Elder & Lubotsky 2009). Still, fail- ing to account for unequally spaced intervals may lead to biased estimation, particularly for young children. Chapter 2 examines the implications of this estimation problem with the help of an often-used dataset for modeling child development.
Chapter 3 draws from the early childhood literature and seeks to determine which skills should be nurtured in early childhood. We know that children start school with different skill levels, and this insight has prompted an interest in early childhood education programs that boost “school readiness” (see, e.g., Clements & Sarama 2011, Diamond & Lee 2011, Dillon et al. 2017, Rege et al. 2021). A challenge in designing these programs is deciding what skills to target, as not all skills are equally important for school success (Duncan et al. 2007, Lewit & Baker 1995). An increasing number of studies have evaluated the effectiveness of early childhood education programs designed to reduce early skill dispari- ties (e.g., Attanasio et al. 2020, Conti et al. 2016, Heckman et al. 2013, Sylvia et al. 2020). These studies generally find that allocating resources to efforts to promote early skill formation can be an effective approach. However, depending on the type of skills targeted and the nature of the intervention, effects may not persist over time. For example, the effects of an investment that targets skills in early childhood that children will eventually develop irrespective of the investment may fade out. As a result, targeting such skills may not be optimal (Bailey et al. 2017).
Chapter 3 studies whether executive functions, defined as the cognitive control processes necessary for concentration and thinking (Diamond & Lee 2011), are skills that programs should focus on. As they involve fun- damental skills, executive functions would appear to be a natural starting point (Diamond & Lee 2011, Howard-Jones et al. 2012). One could argue, however, that the structure provided in school enables children to develop the same level of executive functioning as they would have if they had been the beneficiaries of targeted investments in promoting such executive functions in preschool. But if executive functions are the basis for learning many other skills, children starting school with higher levels of executive functioning may be more efficient at learning other skills than their peers (i.e., skill begets skill). Understanding the processes that drive skill forma- tion in early childhood yields insights for public policy decisions about how educational resources should be used.
Chapter 4 looks at how skills nurtured in early childhood can be maintained through effective education, and examines the literature regarding the education production function. This literature focuses on school inputs that are effective in promoting children’s development. While there are many kinds of school inputs (e.g., class size, number of books, number of computers), Chapter 4 focuses specifically on teachers. Teacher ef- fectiveness may vary widely, even in the same school (Aaronson et al. 2007, Araujo et al. 2016, Jackson 2018, Kraft 2019, Rivkin et al. 2005, Rockoff 2004). Moreover, effective teachers may have long-term impacts on children’s education and labor market outcomes (Chetty et al. 2014b, Opper 2019). Lastly, teachers are the largest budgetary expense in most schools (Hanushek & Rivkin 2006).
While we can identify effective teachers through value-added estimates (Chetty et al. 2014a), we do not know how to replicate them (cf. Kane & Staiger 2012). The literature indicates that a teacher’s readily observable characteristics, such as education, salary, or test scores, do not consistently predict children’s academic achievement (Hanushek & Rivkin 2006). For this reason, researchers have started to focus on what goes on inside the classroom. The child development literature suggests that the quality of the child’s relationship with the teacher and classmates, as perceived by the child, is particularly important (see, e.g., Connell & Wellborn 1991, Hamre & Pianta 2001, Pianta 1997).
Still, the numerous studies that suggest that teacher relationship skills, as perceived by the child, are essential for learning may be biased by a child’s (unobserved) preferences for a particular relationship. Recently, economists have started to refine our measures of investments in education to capture objective, detailed information about the quality of teacher- child interactions (Araujo et al. 2016, Kane et al. 2011). However, these classroom observations are costly and may fail to capture fundamental aspects of a child’s perceptions that ultimately drive behavior (Connell & Wellborn 1991). Moreover, it is important to evaluate teachers and what goes on inside the classroom, with the help of a variety of assessments (Kane & Staiger 2012). Chapter 4 introduces and validates a new approach for measuring teachers’ overall ability to form positive relationships in the classroom (based on the children’s perspectives). This approach has implications for public policy, as such assessments can serve as tools for identifying teachers who need support, promoting development, conduct- ing progress evaluations, and helping policymakers improve quality.
A key motivation for focusing on childhood skill formation is a desire to ensure equal opportunities for all children. Section 2 explains the concept of equality of opportunity and shows that skill disparities emerge early and persist over time. Since all three chapters of this dissertation conceptualize child development in keeping with the technology of skill formation, I introduce this technology and the related literature in Section 3. All chapters address a key challenge – namely, the fact that skills are inherently unobservable. Section 4 elaborates on how, despite that fact, we can learn about a child’s skills. I begin by describing the general intuition underlying the measurement of unobserved variables. Since the measures used in each chapter have been validated in other studies, I briefly describe how such validation is typically achieved. Section 4 concludes with a description of a dedicated measurement model of the type used in each chapter. Section 5 summarizes each of the chapters.
References
Aaronson, D., Barrow, L. & Sander, W. (2007), 'Teachers and student achievement in the Chicago public high schools', Journal of Labor Economics 25(1), 95-135.
https://doi.org/10.1086/508733
Acemoglu, D. & Autor, D. (2011), Skills, tasks and technologies: Implica- tions for employment and earnings, in D. Card & O. Ashenfelter, eds, 'Handbook of Labor Economics', Vol. 4, Chapter 12, pp. 1043-1171.
https://doi.org/10.1016/S0169-7218(11)02410-5
Adler, N. E. & Ostrove, J. M. (1999), Socioeconomic status and health: What we know and what we don't, in 'Annals of the New York Academy of Sciences', Vol. 896, New York Academy of Sciences, pp. 3-15.
https://doi.org/10.1111/j.1749-6632.1999.tb08101.x
Agostinelli, F. & Wiswall, M. (2016), Estimating the technology of chil- dren's skill formation, Working Paper 22442, National Bureau of Eco- nomic Research, Cambridge, Massachusetts.
https://doi.org/10.3386/w22442
Almlund, M., Duckworth, A. L., Heckman, J. & Kautz, T. (2011), Person- ality psychology and economics, in 'Handbook of the Economics of Education', Vol. 4, Elsevier B.V., Chapter 1, pp. 1-181.
https://doi.org/10.1016/B978-0-444-53444-6.00001-8
Almond, D. & Currie, J. (2011), Human capital development before age five, in D. Card & O. Ashenfelter, eds, 'Handbook of Labor Economics', Vol. 4, Chapter 15, pp. 1315-1486.
https://doi.org/10.1016/S0169-7218(11)02413-0
Araujo, M. C., Carneiro, P., Cruz-Aguayo, Y. & Schady, N. (2016), 'Teacher quality and learning outcomes in kindergarten', Quarterly Journal of Economics 131(3), 1415-1453.
https://doi.org/10.1093/qje/qjw016
Attanasio, O., Cattan, S., Fitzsimons, E., Meghir, C. & Rubio-Codina, M. (2020), 'Estimating the production function for human capital: Results from a randomized controlled trial in Colombia', American Economic Review 110(1), 48-85.
https://doi.org/10.1257/aer.20150183
Autor, D. H., Levy, F. & Murnane, R. J. (2003), 'The skill content of recent technological change: An empirical exploration', Quarterly Journal of Economics 118(4), 1279-1333.
https://doi.org/10.1162/003355303322552801
Bailey, D., Duncan, G. J., Odgers, C. L. & Yu, W. (2017), 'Persistence and fadeout in the impacts of child and adolescent interventions', Journal of Research on Educational Effectiveness 10(1), 7-39.
https://doi.org/10.1080/19345747.2016.1232459
Bailey, D. H., Duncan, G. J., Cunha, F., Foorman, B. R. & Yeager, D. S. (2020), 'Persistence and fade-out of educational-intervention effects: Mechanisms and potential solutions', Psychological Science in the Public Interest 21(2), 55-97.
https://doi.org/10.1177/1529100620915848
Baltagi, B. H. & Wu, P. X. (1999), 'Unequally spaced panel data regres- sions with AR(1) disturbances', Econometric Theory 15(6), 814-823.
https://doi.org/10.1017/S0266466699156020
Becker, G. S. (1962), 'Investment in human capital: A theoretical analysis', Journal of Political Economy 70(5), 9-49.
https://doi.org/10.1086/258724
Becker, G. S. (1964), Human Capital: A Theoretical and Empirical Anal- ysis with Special Reference to Education, 3 edn, The University of Chicago Press, Chicago, Illinois.
Becker, G. S. & Tomes, N. (1986), 'Human capital and the rise and fall of families', Journal of Labor Economics 4(3 Pt. 2), 1-47.
https://doi.org/10.1086/298118
Bergstrom, A. R. (1988), 'The history of continuous-time econometric models', Econometric Theory 4(3), 365-383.
https://doi.org/10.1017/S0266466600013359
Blair, C. (2002), 'School readiness: Integrating cognition and emotion in a neurobiological conceptualization of children's functioning at school entry', American Psychologist 57(2), 111-127.
https://doi.org/10.1037/0003-066X.57.2.111
Blau, D. & Currie, J. (2006), Pre-school, day care, and after-school care: Who's minding the kids?, in E. A. Hanushek & F. Welch, eds, 'Handbook of the Economics of Education', Vol. 2, Elsevier B.V., Amsterdam, Netherlands, Chapter 20, pp. 1163-1278.
https://doi.org/10.1016/S1574-0692(06)02020-4
Bollen, K. A. (1989), Structural Equations with Latent Variables, John Wiley & Sons, Inc., New York City, New York.
https://doi.org/10.1002/9781118619179
Borghans, L., Duckworth, A. L., Heckman, J. J. & ter Weel, B. (2008), 'The economics and psychology of personality traits', Journal of Human Resources 43(4), 972-1059.
https://doi.org/10.1353/jhr.2008.0017
https://doi.org/10.3368/jhr.43.4.972
Bradley, R. H. & Caldwell, B. M. (1980), 'The relation of home environ- ment, cognitive competence, and IQ among males and females', Child Development 51(4), 1140-1148.
https://doi.org/10.2307/1129555
Campbell, F., Conti, G., Heckman, J. J., Moon, S. H., Pinto, R., Pungello, E. & Pan, Y. (2014), 'Early childhood investments substantially boost adult health', Science 343(6178), 1478-1485.
https://doi.org/10.1126/science.1248429
Center for Human Resource Research (2009), NLSY79 Child and Young Adult Data User's Guide, Center for Human Resource Research, Ohio State University, Columbus, Ohio. URL: https://search.library.wisc.edu/catalog/9910094201202121
Chapman, J. W. & Tunmer, W. E. (1995), 'Development of young chil- dren's reading self-concepts: An examination of emerging subcom- ponents and their relationship with reading achievement', Journal of Educational Psychology 87(1), 154-167.
https://doi.org/10.1037/0022-0663.87.1.154
Chapman, J. W., Tunmer, W. E. & Prochnow, J. E. (2000), 'Early reading- related skills and performance, reading self-concept, and the devel- opment of academic self-concept: A longitudinal study', Journal of Educational Psychology 92(4), 703-708.
https://doi.org/10.1037/0022-0663.92.4.703
Chetty, R., Friedman, J. N. & Rockoff, J. E. (2014a), 'Measuring the impacts of teachers I: Evaluating bias in teacher value-added estimates', American Economic Review 104(9), 2593-2632.
https://doi.org/10.1257/aer.104.9.2593
Chetty, R., Friedman, J. N. & Rockoff, J. E. (2014b), 'Measuring the impacts of teachers II: Teacher value-added and student outcomes in adulthood', American Economic Review 104(9), 2633-2679.
https://doi.org/10.1257/aer.104.9.2633
Chetty, R., Hendren, N. & Katz, L. F. (2016), 'The effects of exposure to better neighborhoods on children: New evidence from the moving to opportunity experiment', American Economic Review 106(4), 855-902.
https://doi.org/10.1257/aer.20150572
Clements, D. H. & Sarama, J. (2011), 'Early childhood mathematics intervention', Science 333(6045), 968-970.
https://doi.org/10.1126/science.1204537
Connell, J. P. & Wellborn, J. G. (1991), Competence, Autonomy, and Relatedness: A Motivational Analysis of Self-System Processes, The Minnesota Symposia on Child Psychology, Volume 23., Lawrence Erl- baum Associates, Inc, Hillsdale, New Jersey, pp. 43-77.
Conti, G., Heckman, J. J. & Pinto, R. (2016), 'The effects of two influ- ential early childhood interventions on health and healthy behaviour', Economic Journal 126(596), F28-F65.
https://doi.org/10.1111/ecoj.12420
Crawford, C., Dearden, L. & Meghir, C. (2010), When you are born matters: The impact of date of birth on educational outcomes in England, Working Paper 10,06, Institute for Fiscal Studies, London, England.
https://doi.org/10.1920/wp.ifs.2010.1006
Cunha, F. & Heckman, J. (2007), 'The technology of skill formation', American Economic Review 97(2), 31-47.
https://doi.org/10.1257/aer.97.2.31
Cunha, F. & Heckman, J. J. (2008), 'Formulating, identifying and esti- mating the technology of cognitive and noncognitive skill formation', Journal of Human Resources 43(4), 738-782.
https://doi.org/10.1353/jhr.2008.0019
https://doi.org/10.3368/jhr.43.4.738
Cunha, F. & Heckman, J. J. (2009), 'The economics and psychology of inequality and human development', Journal of the European Economic Association 7(2-3), 320-364.
https://doi.org/10.1162/JEEA.2009.7.2-3.320
Cunha, F., Heckman, J. J., Lochner, L. & Masterov, D. V. (2006), Inter- preting the evidence on life cycle skill formation, in E. A. Hanushek & F. Welch, eds, 'Handbook of the Economics of Education', Vol. 1, Elsevier B.V., Amsterdam, Netherlands, Chapter 12, pp. 697-812.
https://doi.org/10.1016/S1574-0692(06)01012-9
Cunha, F., Heckman, J. J. & Schennach, S. M. (2010), 'Estimating the tech- nology of cognitive and noncognitive skill formation', Econometrica 78(3), 883-931.
https://doi.org/10.3982/ECTA6551
Cunha, F., Nielsen, E. & Williams, B. (2021), 'The econometrics of early childhood human capital and investments', Annual Review of Economics 13, 487-513.
https://doi.org/10.1146/annurev-economics-080217-053409
Diamond, A. & Lee, K. (2011), 'Interventions shown to aid execu- tive function development in children 4 to 12 years old', Science 333(6045), 959-964.
https://doi.org/10.1126/science.1204529
Dillon, M. R., Kannan, H., Dean, J. T., Spelke, E. S. & Duflo, E. (2017), 'Cognitive science in the field: A preschool intervention durably en- hances intuitive but not formal mathematics', Science 357(6346), 47-55.
https://doi.org/10.1126/science.aal4724
Duncan, G. J., Dowsett, C. J., Claessens, A., Magnuson, K., Huston, A. C., Klebanov, P., Pagani, L. S., Feinstein, L., Engel, M., Brooks-Gunn, J., Sexton, H., Duckworth, K. & Japel, C. (2007), 'School readiness and later achievement', Developmental Psychology 43(6), 1428-1446.
https://doi.org/10.1037/0012-1649.43.6.1428
Duncan, G. J. & Murnane, R. J. (2011), Whither Opportunity? Rising Inequality, Schools, and Children's Life Chances, Russell Sage Founda- tion, New York City, New York.
Elder, T. E. & Lubotsky, D. H. (2009), 'Kindergarten entrance age and children's achievement: Impacts of state policies, family background, peers', Journal of Human Resources 44(3), 641-683.
https://doi.org/10.1353/jhr.2009.0015
Fredriksson, P., Öckert, B. & Oosterbeek, H. (2010), Inside the black box of class size: Mechanisms, behavioral responses, and social background, Working Paper 8019, Institute for the Study of Labor, Bonn, Germany.
Goldhaber, D. & Hansen, M. (2010), 'Using performance on the job to inform teacher tenure decisions', American Economic Review 100(2), 250-255.
https://doi.org/10.1257/aer.100.2.250
Hamre, B. K. & Pianta, R. C. (2001), 'Early teacher-child relationships and the trajectory of children's school outcomes through eighth grade', Child Development 72(2), 625-638.
https://doi.org/10.1111/1467-8624.00301
Hansen, K. T., Heckman, J. J. & Mullen, K. J. (2004), 'The effect of school- ing and ability on achievement test scores', Journal of Econometrics 121(1-2), 39-98.
https://doi.org/10.1016/j.jeconom.2003.10.011
Hanushek, E. A. & Rivkin, S. G. (2006), Teacher quality, in E. A. Hanushek & F. Welch, eds, 'Handbook of the Economics of Education', Vol. 2, Elsevier B.V., Amsterdam, Netherlands, Chapter 18, pp. 1051-1078.
https://doi.org/10.1016/S1574-0692(06)02018-6
Hanushek, E. A. & Rivkin, S. G. (2010), 'Generalizations about using value-added measures of teacher quality', American Economic Review 100(2), 267-271.
https://doi.org/10.1257/aer.100.2.267
Heckman, J. J. & Mosso, S. (2014), 'The economics of human development and social mobility', Annual Review of Economics 6, 689-733.
https://doi.org/10.1146/annurev-economics-080213-040753
Heckman, J., Pinto, R. & Savelyev, P. (2013), 'Understanding the mecha- nisms through which an influential early childhood program boosted adult outcomes', American Economic Review 103(6), 2052-2086.
https://doi.org/10.1257/aer.103.6.2052
Howard-Jones, P. A., Washbrook, E. V. & Meadows, S. (2012), 'The timing of educational investment: A neuroscientific perspective', Devel- opmental Cognitive Neuroscience 25, S18-S29.
https://doi.org/10.1016/j.dcn.2011.11.002
Iversen, J. M. V. & Bonesrønning, H. (2013), 'Disadvantaged students in the early grades: Will smaller classes help them?', Education Economics 21(4), 305-324.
https://doi.org/10.1080/09645292.2011.623380
Jackson, C. K. (2018), 'What do test scores miss? the importance of teacher effects on non-test score outcomes', Journal of Political Econ- omy 126(5), 2072-2107.
https://doi.org/10.1086/699018
Jacob, B. & Rothstein, J. (2016), 'The measurement of student ability in modern assessment systems', Journal of Economic Perspectives 30(3), 85-108.
https://doi.org/10.1257/jep.30.3.85
Jones, R. H. & Boadi-Boateng, F. (1991), 'Unequally spaced longitudinal data with AR(1) serial correlation', Biometrics 47(1), 161-175.
https://doi.org/10.2307/2532504
Kane, T. J. & Staiger, D. O. (2012), Gathering feedback for teaching: Com- bining high-quality observations with student surveys and achievement gains, Report.
Kane, T. J., Taylor, E. S., Tyler, J. H. & Wooten, A. L. (2011), 'Identifying effective classroom practices using student achievement data', Journal of Human Resources 46(3), 587-613.
https://doi.org/10.1353/jhr.2011.0010
https://doi.org/10.3368/jhr.46.3.587
Klausen, T. & Reikerås, E. (2016), Regnefaktaprøven [Assessment of Arithmetic Facts], Norwegian Reading Centre, Stavanger, Norway.
Kotlarski, I. (1967), 'On characterizing the gamma and the normal distri- bution', Pacific Journal of Mathematics 20(1), 69-76.
https://doi.org/10.2140/pjm.1967.20.69
Kraft, M. A. (2019), 'Teacher effects on complex cognitive skills and social-emotional competencies', Journal of Human Resources 54(1), 1-36.
https://doi.org/10.3368/jhr.54.1.0916.8265R3
Lewit, E. M. & Baker, L. S. (1995), 'School readiness', The Future of children / Center for the Future of Children, the David and Lucile Packard Foundation 5(2), 128-139.
https://doi.org/10.2307/1602361
Madansky, A. (1964), 'Instrumental variables in factor analysis', Psy- chometrika 29(2), 105-113.
https://doi.org/10.1007/BF02289693
Masten, A. S. & Coatsworth, J. D. (1998), 'The development of compe- tence in favorable and unfavorable environments: Lessons from research on successful children', American Psychologist 53(2), 205-220.
https://doi.org/10.1037/0003-066X.53.2.205
McKenzie, D. J. (2001), 'Estimation of AR(1) models with unequally spaced pseudo-panels', The Econometrics Journal 4(1), 89-108.
https://doi.org/10.1111/1368-423X.00058
Millimet, D. L. & McDonough, I. K. (2017), 'Dynamic panel data models with irregular spacing: With an application to early childhood develop- ment', Journal of Applied Econometrics 32(4), 725-743.
https://doi.org/10.1002/jae.2548
National Research Council Institute of Medicine (2000), From neurons to neighborhoods: The science of early childhood development, National Academy Press, Washington, D.C.
OECD (2021), OECD Skills Outlook 2021, OECD Publishing, Paris, France.
Opper, I. M. (2019), 'Does helping John help Sue? evidence of spillovers in education', American Economic Review 109(3), 1080-1115.
https://doi.org/10.1257/aer.20161226
Parker, J. G. & Asher, S. R. (1987), 'Peer relations and later personal adjustment: Are low-accepted children at risk?', Psychological Bulletin 102(3), 357-389.
https://doi.org/10.1037/0033-2909.102.3.357
Peterson, J. L. & Zill, N. (1986), 'Marital disruption, parent-child relation- ships, and behavior problems in children', Journal of Marriage and the Family pp. 295-307.
https://doi.org/10.2307/352397
Pianta, R. C. (1997), 'Adult-child relationship processes and early school- ing', Early Education and Development 8(1), 11-26.
https://doi.org/10.1207/s15566935eed0801_2
Rege, M., Størksen, I., Solli, I. F., Kalil, A., McClelland, M. M., ten Braak, D., Lenes, R., Lunde, S., Breive, S., Carlsen, M., Erfjord, I. & Hundeland, P. S. (2021), 'The effects of a structured curriculum on preschool effectiveness: A field experiment', Journal of Human Resources .
https://doi.org/10.3368/jhr.0220-10749R3
Rivkin, S. G., Hanushek, E. A. & Kain, J. F. (2005), 'Teachers, schools, and academic achievement', Econometrica 73(2), 417-458.
https://doi.org/10.1111/j.1468-0262.2005.00584.x
Rockoff, J. E. (2004), 'The impact of individual teachers on student achievement: Evidence from panel data', American Economic Review 94(2), 247-252.
https://doi.org/10.1257/0002828041302244
Roemer, J. E. (2000), Equality of Opportunity, Harvard University Press, Cambridge, Massachusetts.
https://doi.org/10.1515/9780691190334-004
Rosner, B. & Muǹoz, A. (1988), 'Autoregressive modelling for the analysis of longitudinal data with unequally spaced examinations', Statistics in Medicine 7(1-2), 59-71.
https://doi.org/10.1002/sim.4780070110
Sasaki, Y. & Xin, Y. (2017), 'Unequal spacing in dynamic panel data: Iden- tification and estimation', Journal of Econometrics 196(2), 320-330.
https://doi.org/10.1016/j.jeconom.2016.10.002
Schanzenbach, D. W. (2006), 'What have researchers learned from Project STAR', Brookings Paper on Education Policy (9), 205-228.
https://doi.org/10.1353/pep.2007.0007
Skrondal, A. & Rabe-Hesketh, S. (2004), Generalized Latent Variable Modeling: Multilevel, Longitudinal, and Structural Equation Models, Interdisciplinary Statistics, 1 edn, Chapman & Hall/CRC, Boca Raton, Florida.
https://doi.org/10.1201/9780203489437
Spitz-Oener, A. (2006), 'Technical change, job tasks, and rising educa- tional demands: Looking outside the wage structure', Journal of Labor Economics 24(2), 235-270.
https://doi.org/10.1086/499972
Størksen, I. & Mosvold, R. (2013), 'Assessing early math skills with tablet computers: Development of the ani banani math test (ABMT) for young children [Utdanning2020]'.
Sylvia, S., Warrinnier, N., Luo, R., Yue, A., Attanasio, O., Medina, A. & Rozelle, S. (2020), 'From quantity to quality: Delivering a home- based parenting intervention through China's family planning cadres', Economic Journal 131(635), 1365-1400.
https://doi.org/10.1093/ej/ueaa114
ten Braak, D. & Størksen, I. (2021), 'Psychometric properties of the Ani Banani Math Test', European Journal of Developmental Psychology 18(4), 610-628.
https://doi.org/10.1080/17405629.2021.1879046
Todd, P. E. & Wolpin, K. I. (2003), 'On the specification and estimation of the production function for cognitive achievement', Economic Journal 113(485), F3-F33.
https://doi.org/10.1111/1468-0297.00097
Torgerson, W. S. (1958), Theory and Methods of Scaling, John Wiley & Sons, Inc., New York City, New York.
VandenBos, G. R. E. (2007), APA Dictionary of Psychology, APA Dictio- nary of Psychology, American Psychological Association, Washington, D.C.
Voelkle, M. C. & Oud, J. H. L. (2013), 'Continuous time modelling with individually varying time intervals for oscillating and non-oscillating processes', British Journal of Mathematical and Statistical Psychology 66(1), 103-126.
https://doi.org/10.1111/j.2044-8317.2012.02043.x
Vygotsky, L. S. (1978), Mind in Society: The Development of Higher Psychological Processes, Harvard University Press, Cambridge, Mas- sachusetts.
Wansbeek, T. & Meijer, E. (2000), Measurement Error and Latent Vari- ables in Econometrics, Advanced Textbooks in Economics, Elsevier Science B.V., Amsterdam, Netherlands.
Williams, B. (2020), 'Identification of the linear factor model', Economet- ric Reviews 39(1), 92-109.
https://doi.org/10.1080/07474938.2018.1550042
Chapter 2:
Agostinelli, F. & Wiswall, M. (2016), Estimating the technology of children's skill formation, Working Paper 22442, National Bureau of Eco- nomic Research, Cambridge, Massachusetts.
https://doi.org/10.3386/w22442
Alwin, D. F. (1989), 'Problems in the estimation and interpretation of the reliability of survey data', Quality and Quantity 23(3), 277-331.
https://doi.org/10.1007/BF00172447
Anderson, T. W. (1957), 'Maximum likelihood estimates for a multivariate normal distribution when some observations are missing', Journal of the American Statistical Association 52(278), 200-203.
https://doi.org/10.1080/01621459.1957.10501379
Anderson, T. W. & Rubin, H. (1956), Statistical Inference in Factor Anal- ysis, Vol. 5 of Proceedings of the Third Berkeley Symposium on Mathe- matical Statistics and Probability, Volume 5: Contributions to Econo- metrics, Industrial Research, and Psychometry, University of California Press, Berkeley, California.
Arnold, L. (1974), Stochastic Differential Equations: Theory and Appli- cations, John Wiley & Sons, Inc., New York City, New York.
Attanasio, O., Cattan, S., Fitzsimons, E., Meghir, C. & Rubio-Codina, M. (2020), 'Estimating the production function for human capital: Results from a randomized controlled trial in Colombia', American Economic Review 110(1), 48-85.
https://doi.org/10.1257/aer.20150183
Attanasio, O., Meghir, C. & Nix, E. (2020), 'Human capital develop- ment and parental investment in india', Review of Economic Studies 87(6), 2511-2541.
https://doi.org/10.1093/restud/rdaa026
Balestra, P. & Nerlove, M. (1966), 'Pooling cross section and time series data in the estimation of a dynamic model: The demand for natural gas', Econometrica 34(3), 585-612.
https://doi.org/10.2307/1909771
Baltagi, B. H. & Wu, P. X. (1999), 'Unequally spaced panel data regres- sions with AR(1) disturbances', Econometric Theory 15(6), 814-823.
https://doi.org/10.1017/S0266466699156020
Becker, G. S. & Tomes, N. (1976), 'Child endowments and the quantity and quality of children', Journal of Political Economy 84(4), S143-S162.
https://doi.org/10.1086/260536
Ben-Moshe, D. (2012), Essays on Nonparametric Identification, Doctoral thesis.
Ben-Moshe, D. (2018), 'Identification of joint distributions in dependent factor models', Econometric Theory 34(1), 134-165.
https://doi.org/10.1017/S026646661700007X
Bergstrom, A. R. (1988), 'The history of continuous-time econometric models', Econometric Theory 4(3), 365-383.
https://doi.org/10.1017/S0266466600013359
Bollen, K. A. (1989), Structural Equations with Latent Variables, John Wiley & Sons, Inc., New York City, New York.
https://doi.org/10.1002/9781118619179
Borghans, L., Duckworth, A. L., Heckman, J. J. & ter Weel, B. (2008), 'The economics and psychology of personality traits', Journal of Human Resources 43(4), 972-1059.
https://doi.org/10.1353/jhr.2008.0017
https://doi.org/10.3368/jhr.43.4.972
Bradley, R. H. & Caldwell, B. M. (1980), 'The relation of home environ- ment, cognitive competence, and IQ among males and females', Child Development 51(4), 1140-1148.
https://doi.org/10.2307/1129555
Bradley, R. H. & Caldwell, B. M. (1984), 'The relation of infants' home environments to achievement test performance in first grade: A follow- up study', Child Development 55(3), 803-809.
https://doi.org/10.1111/j.1467-8624.1984.tb03817.x
Cawley, J., Heckman, J. & Vytlacil, E. (2001), 'Three observa- tions on wages and measured cognitive ability', Labour Economics 8(4), 419-442.
https://doi.org/10.1016/S0927-5371(01)00039-2
Center for Human Resource Research (2009), NLSY79 Child and Young Adult Data User's Guide, Center for Human Resource Research, Ohio State University, Columbus, Ohio. URL: https://search.library.wisc.edu/catalog/9910094201202121
Crawford, C., Dearden, L. & Meghir, C. (2010), When you are born matters: The impact of date of birth on educational outcomes in England, Working Paper 10,06, Institute for Fiscal Studies, London, England.
https://doi.org/10.1920/wp.ifs.2010.1006
Cunha, F. & Heckman, J. (2007), 'The technology of skill formation', American Economic Review 97(2), 31-47.
https://doi.org/10.1257/aer.97.2.31
Cunha, F. & Heckman, J. J. (2008), 'Formulating, identifying and esti- mating the technology of cognitive and noncognitive skill formation', Journal of Human Resources 43(4), 738-782.
https://doi.org/10.1353/jhr.2008.0019
https://doi.org/10.3368/jhr.43.4.738
Cunha, F., Heckman, J. J. & Schennach, S. M. (2010), 'Estimating the tech- nology of cognitive and noncognitive skill formation', Econometrica 78(3), 883-931.
https://doi.org/10.3982/ECTA6551
Cunha, F., Nielsen, E. & Williams, B. (2021), 'The econometrics of early childhood human capital and investments', Annual Review of Economics 13, 487-513.
https://doi.org/10.1146/annurev-economics-080217-053409
Del Boca, D., Flinn, C. & Wiswall, M. (2014), 'Household choices and child development', Review of Economic Studies 81(1), 137-185.
https://doi.org/10.1093/restud/rdt026
Driver, C. C. Voelkle, M. C. (2018), 'Hierarchical bayesian continuous time dynamic modeling.', Psychological Methods 23(4), 774.
https://doi.org/10.1037/met0000168
Elder, T. E. & Lubotsky, D. H. (2009), 'Kindergarten entrance age and children's achievement: Impacts of state policies, family background, peers', Journal of Human Resources 44(3), 641-683.
https://doi.org/10.1353/jhr.2009.0015
Hamerle, A., Nagl, W. & Singer, H. (1991), 'Problems with the estimation of stochastic differential equations using structural equations models', The Journal of Mathematical Sociology 16(3), 201-220.
https://doi.org/10.1080/0022250X.1991.9990088
Hamerle, A., Singer, H. & Nagl, W. (1993), 'Identification and estimation of continuous time dynamic systems with exogenous variables using panel data', Econometric Theory 9(2), 283-295.
https://doi.org/10.1017/S0266466600007544
Heckman, J. J. & Mosso, S. (2014), 'The economics of human development and social mobility', Annual Review of Economics 6, 689-733.
https://doi.org/10.1146/annurev-economics-080213-040753
Heckman, J., Pinto, R. & Savelyev, P. (2013), 'Understanding the mecha- nisms through which an influential early childhood program boosted adult outcomes', American Economic Review 103(6), 2052-2086.
https://doi.org/10.1257/aer.103.6.2052
Holzinger, K. J. & Swineford, F. (1937), 'The bi-factor method', Psy- chometrika 2(1), 41-54.
https://doi.org/10.1007/BF02287965
Hu, Y. & Schennach, S. M. (2008), 'Instrumental variable treatment of nonclassical measurement error models', Econometrica 76(1), 195-216.
https://doi.org/10.1111/j.0012-9682.2008.00823.x
Jones, R. H. & Boadi-Boateng, F. (1991), 'Unequally spaced longitudinal data with AR(1) serial correlation', Biometrics 47(1), 161-175.
https://doi.org/10.2307/2532504
Kalil, A. (2015), Inequaliy Begins at Home: The Role of Parenting in the Diverging Destinies of Rich and Poor Children, Vol. 5 of National Symposium on Family Issues, 1 edn, Springer International Publishing, Cham, Germany, book section 5, pp. 63-82.
https://doi.org/10.1007/978-3-319-08308-7_5
Kotlarski, I. (1967), 'On characterizing the gamma and the normal distri- bution', Pacific Journal of Mathematics 20(1), 69-76.
https://doi.org/10.2140/pjm.1967.20.69
Li, T. & Vuong, Q. (1998), 'Nonparametric estimation of the measurement error model using multiple indicators', Journal of Multivariate Analysis 65(2), 139-165.
https://doi.org/10.1006/jmva.1998.1741
Little, R. J. A. & Rubin, D. B. (2002), Statistical Analysis with Missing Data, Wiley Series in Probability and Statistics, 2 edn, John Wiley & Sons, Inc., Hoboken, New Jersey.
McArdle, J. J. & McDonald, R. P. (1984), 'Some algebraic properties of the reticular action model for moment structures', British Journal of Mathematical and Statistical Psychology 37(2), 234-251.
https://doi.org/10.1111/j.2044-8317.1984.tb00802.x
McKenzie, D. J. (2001), 'Estimation of AR(1) models with unequally spaced pseudo-panels', The Econometrics Journal 4(1), 89-108.
https://doi.org/10.1111/1368-423X.00058
Meredith, W. (1993), 'Measurement invariance, factor analysis and facto- rial invariance', Psychometrika 58(4), 525-543.
https://doi.org/10.1007/BF02294825
Millimet, D. L. & McDonough, I. K. (2017), 'Dynamic panel data models with irregular spacing: With an application to early childhood develop- ment', Journal of Applied Econometrics 32(4), 725-743.
https://doi.org/10.1002/jae.2548
Moler, C. & Van Loan, C. (2003), 'Nineteen dubious ways to compute the exponential of a matrix, twenty-five years later', SIAM review 45(1), 3-49.
https://doi.org/10.1137/S00361445024180
National Research Council Institute of Medicine (2000), From neurons to neighborhoods: The science of early childhood development, National Academy Press, Washington, D.C.
Neale, M. C., Hunter, M. D., Pritikin, J. N., Zahery, M., Brick, T. R., Kirkpatrick, R. M., Estabrook, R., Bates, T. C., Maes, H. H. & Boker, S. M. (2016), 'OpenMx 2.0: Extended structural equation and statistical modeling', Psychometrika 81(2), 535-549.
https://doi.org/10.1007/s11336-014-9435-8
Oud, J. H. L. & Jansen, R. A. R. G. (2000), 'Continuous time state space modeling of panel data by means of SEM', Psychometrika 65(2), 199-215.
https://doi.org/10.1007/BF02294374
Rege, M., Størksen, I., Solli, I. F., Kalil, A., McClelland, M. M., ten Braak, D., Lenes, R., Lunde, S., Breive, S., Carlsen, M., Erfjord, I. & Hundeland, P. S. (2021), 'The effects of a structured curriculum on preschool effectiveness: A field experiment', Journal of Human Resources .
https://doi.org/10.3368/jhr.0220-10749R3
Rosner, B. & Muǹoz, A. (1988), 'Autoregressive modelling for the analysis of longitudinal data with unequally spaced examinations', Statistics in Medicine 7(1-2), 59-71.
https://doi.org/10.1002/sim.4780070110
Sasaki, Y. & Xin, Y. (2017), 'Unequal spacing in dynamic panel data: Iden- tification and estimation', Journal of Econometrics 196(2), 320-330.
https://doi.org/10.1016/j.jeconom.2016.10.002
Schennach, S. M. (2004a), 'Estimation of nonlinear models with measure- ment error', Econometrica 72(1), 33-75.
https://doi.org/10.1111/j.1468-0262.2004.00477.x
Schennach, S. M. (2004b), 'Nonparametric regression in the presence of measurement error', Econometric Theory 20(6), 1046-1093.
https://doi.org/10.1017/S0266466604206028
Singer, H. (1990), Parameterschätzung in Zeitkontinuierlichen Dynamis- chen Systemen, Doctoral thesis.
Singer, H. (1993), 'Continuous-time dynamical systems with sampled data, errors of measurement and unobserved components', Journal of Time Series Analysis 14(5), 527-545.
https://doi.org/10.1111/j.1467-9892.1993.tb00162.x
Singer, H. (1995), 'Analytical score function for irregularly sampled con- tinuous time stochastic processes with control variables and missing values', Econometric Theory 11(4), 721-735.
https://doi.org/10.1017/S0266466600009701
Skrondal, A. & Laake, P. (2001), 'Regression among factor scores', Psy- chometrika 66(4), 563-575.
https://doi.org/10.1007/BF02296196
Skrondal, A. & Rabe-Hesketh, S. (2004), Generalized Latent Variable Modeling: Multilevel, Longitudinal, and Structural Equation Models, Interdisciplinary Statistics, 1 edn, Chapman & Hall/CRC, Boca Raton, Florida.
https://doi.org/10.1201/9780203489437
Sylvia, S., Warrinnier, N., Luo, R., Yue, A., Attanasio, O., Medina, A. & Rozelle, S. (2020), 'From quantity to quality: Delivering a home- based parenting intervention through China's family planning cadres', Economic Journal 131(635), 1365-1400.
https://doi.org/10.1093/ej/ueaa114
Thijssen, M. W. P., Rege, M., Solli, I. F. & Størksen, I. (2022), Cross- productivities of executive functions.
Todd, P. E. & Wolpin, K. I. (2003), 'On the specification and estimation of the production function for cognitive achievement', Economic Journal 113(485), F3-F33.
https://doi.org/10.1111/1468-0297.00097
Todd, P. E. & Wolpin, K. I. (2007), 'The production of cognitive achieve- ment in children: Home, school, and racial test score gaps', Journal of Human capital 1(1), 91-136.
https://doi.org/10.1086/526401
Torgerson, W. S. (1958), Theory and Methods of Scaling, John Wiley & Sons, Inc., New York City, New York.
U.S. Department of Defense (1982), Profile of American Youth: 1980 Nationwide Administration of the Armed Services Vocational Aptitude Battery, Office of the Assistant Secretary of Defense for Manpower, Reserve Affairs, and Logistics, Washington, D.C.
Voelkle, M. C. & Oud, J. H. L. (2013), 'Continuous time modelling with individually varying time intervals for oscillating and non-oscillating processes', British Journal of Mathematical and Statistical Psychology 66(1), 103-126.
https://doi.org/10.1111/j.2044-8317.2012.02043.x
Zahery, M., Maes, H. H. & Neale, M. C. (2017), 'CSOLNP: Numerical optimization engine for solving non-linearly constrained problems', Twin Research and Human Genetics 20(4), 290-297.
https://doi.org/10.1017/thg.2017.28
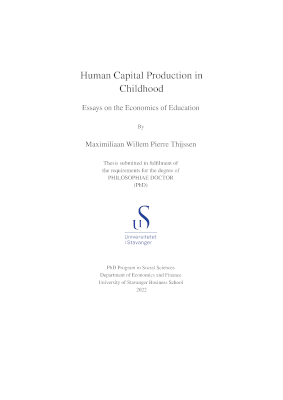